Dinakar Munagala, co-founder and CEO, Blaize, believes his Graph Streaming Processing (GSP) architecture stands a chance to infiltrate the former camp. Blaize seeks to fill gaps left open by edge AI solutions currently available.
AI processing is changing the world order among CPU, GPU, and FPGA companies, with a host of AI processor startups joining the fray. The fight was once mostly in data centers, but they’ve all had to decamp to a new battlefield at the network edge. Driven by that premise, Blaize, an AI processor startup in El Dorado Hills, Calif., is heading straight to the edge with its just-announced AI hardware and software.
The market forces sending AI inference to the edge are well understood. Privacy concerns, bandwidth issues (going back and forth between edge to cloud), latency and cost worries drive AI processing more and more edgeward.
Less understood, however, is if the industry will see a nouvelle AI processing architecture sweep the edge AI market. Or, will there be a slew of SoCs and AI accelerators gunning for different segments on the edge?
Dinakar Munagala, co-founder and CEO, Blaize, believes his Graph Streaming Processing (GSP) architecture stands a chance to infiltrate the former camp. Blaize seeks to fill gaps left open by edge AI solutions currently available.
Different applications require power consumption, latency, processor performance, and programmability in different measures (the balance affects costs, of course). It’s difficult to cover all the possibilities, and that creates the gaps Blaize intend to occupy.
A gulf exists, he stressed, between so-called edge AI solutions whose computing power is often too small, and legacy GPUs and CPUs which are too expensive and too power hungry at the edge.
New products
Blaize is not one of those all-talk-no-product startups. It taped out a test chip a year ago. Its production modules have been up and running since early July.
Since then the company has already put its test modules in the hands of “well over half a dozen potential customers,” according to Munagala. Lead investor and partner Denso has helped Blaize advance its GSP architecture in the automotive field. Given that many customers have been playing with Blaize’s hardware and software for a year, Blaize is confident that its GSP will be designed into industrial, automotive, retail stories and factory floors on the edge.
Meanwhile, Blaize last week unveiled two platforms — Pathfinder and Xplorer. Both will become available in the fourth quarter.
Munagala emphasized that his company isn’t in the business of selling chips. It offers modules and plug-in cards.
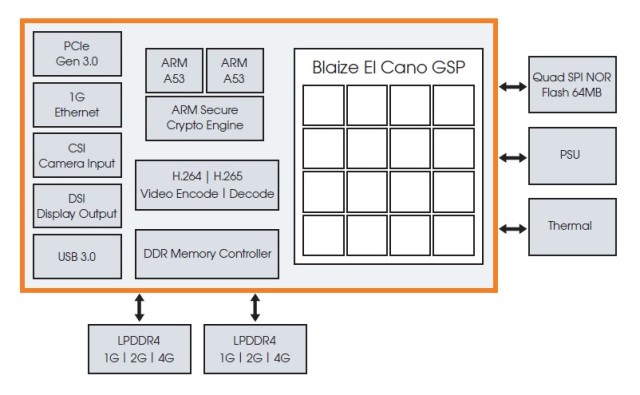
What’s inside P1600? 16 El Cano GSP cores, dual ARM A53 cores, H.264/H.265 video decode, H.264/H.265 video encode, multiple embedded interfaces to camera, display, ethernet, PCIe and USB. (Image: Blaize)
The first product on the Pathfinder platform is the P1600 embedded system-on-module, priced at $399 in volume. The credit card-size module, integrated with Arm CPU and Blaize GSP, is a standalone system — without a host processor — that can go inside cameras, for example.
Blaize also rolled out two AI accelerators, the X1600E and X1600P, both designed for the edge of the enterprise, built on its Xplorer platform. The X1600E, at $299 in volume, is a miniature accelerator platform for small and power-constrained environments such as convenience stores or industrial sites, Blaize explained. It can be easily added “to accelerate AI apps in industrial PCs or as a rack of cards in a small one rack unit server.”
The X1600P, available in volume for $999, is a standard PCIe-based accelerator. The X1600P can “replace a power-hungry desktop GPU in edge servers and provide anywhere from 16-64TOPS of AI inference performance” at very low power, according to Blaize.
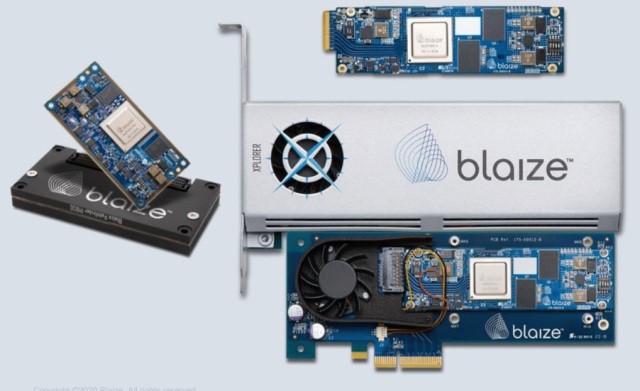
Blaize new product lineup: P1600 (left), X1600E (top right), X1600P (top bottom). (Image: Blaize)
How does Blaize GSP stack up?
Blaize describes its GSP as follows:
With 16 GSP cores and 16TOPS of AI inference performance within a tiny 7W power envelope, GSP delivers up to 60x better system-level efficiency vs. GPU/CPUs for edge AI applications.
Sixteen tera operations per second (TOPS) at 7 watts sounds really low-power. Many tech analysts are, however, frustrated that Blaize is comparing its GSP with GPU/CPU, but not with other AI accelerators popping up on the edge AI market.
How does GSP stack up against the others?
Aditya Kaul, a research director at Omdia, told EE Times, “That’s a good question. For the most part, it’s hard to compare solutions, as most use varying metrics from TOPS, FLOPS at different resolutions like INT8, FP32 etc.”
He added, “The MLPerf benchmark is the only true comparison that I know of — however most of that is for datacenter rather than edge.” Kaul said Omdia plans to benchmark vendors in the AI datacenter and AI edge market in 2021. He promised that around the second quarter next year, that study should include some technology comparisons apart from commercial and business metrics.
Karl Freund, senior analyst, HPC and Deep Learning at Moor Insights & Strategy, offered a broad-brush review. “Yes, Blaize does look strong and ahead of others such as Groq and Intel (Habana). It is a low-power solution, targeting areas below Nvidia Xavier and Jetson.” Freund added, “It will take some time before we will have in-depth comparisons.” Pressed to compare Blaize’s solution with Nvidia’s Xavier, Freund said, “Well, the Xavier is higher cost and power, but with many different types of processors, including CPU, GPU, DLA, etc. Blaize is lower on all three.”
Which Blaize edge apps will shine?
There are several things going for Blaize.
For starters, its GSP architecture offers a “low latency, low power consumption and programmability” that other AI processors can’t provide. As the new class of data-driven AI workloads constantly evolve, GSP’s ability to provide full programmability is vital.
Low power is an absolute mandate, because the solution must reside at the edge. Equally significant is compute processing performance.
Consider GSP applications on the factory floor. Cameras are in place to reduce injuries from human/robot interactions. The cameras also monitor product quality and count.
Blaize’s Pathfinder P1600, teamed with five HD Power-over-Ethernet cameras, demonstrated that it can provide five independent neural networks running at 10 frames per second (50 FPS total) with less than 100ms latency, Blaize claimed. The P1600 performs functions that include the monitoring pose and position of human operators and robots, spotting product IDs and counting products while enhancing quality, safety and security.
Most intriguing, noted Omdia’s Kaul, was the GSP’s capabilities “supporting multiple workloads simultaneously across different camera streams,” enabling sensor fusion, object detection, image noise reduction, Lidar and object detection.
Similarly, applied to sensor fusion, a single Blaize Pathfinder P1600 can handle tasks that range from ISP processing and segmentation (running SwiftNet) to fusing video and lidar/radar data, Munagala explained. “We can do high resolution FHD video and Lidar/Radar sensor fusion application running as a complete graph-native application.”
Freund noted, “Embedded apps like smart cameras are ideal for this chip. And, instead of having an on-board Arm CPU, programmers can develop using data flow (graph) algorithms.”
Munagala stressed that all the modules Blaize is rolling out are already “industrial-grade.”
What about software?
If Blaize hopes to claim a big piece of the edge AI market, nothing is more important than a software suite that helps developers address the vast AI applications crowding the edge.
Blaize announced that its AI software offers tools to both traditional developers and non-coders. While training AI models cloud-service data centers are well understood, the industry has grown increasingly aware of how hard it is to move those models to the edge. Blaize’s new tool sets, such as Blaize Netdelopy, help developers prune, quantify and optimize edge-aware algorithms to improve accuracy and performance.
Blaize also rolled out “AI Studio,” designed to enable “code-free” development of edge applications, complementing the updated Picasso software suite.
During his interview with EE Times, Munagala said, “Think about pineapple plantations in the Philippines. They are considering using AI to improve their crop and supply chain of their produce.”
In reality, such a lofty aspiration is hard to achieve by pineapple growers with no in-house data scientists or AI developers.
Similarly, factory managers dream of applying AI to improve the productivity and safety of their factory floors. Again, there’s a huge gap between ambition and reality. They know where the problems lie, but many don’t even know where to start.
With its newly launched AI Studio, Blaize is promising “a code-free platform” for domain experts to create and complete AI product solutions.
As Freund put it, “Some edge solutions are low power and low cost but have low performance. Google Edge TPU, for example.” Edge TPU is an ASIC designed to run AI at the edge. He added, “As Blaize rightly points out, GSP strikes a better balance between performance, cost, and power.”
GSP is the right architecture?
Finally, the nagging question: Is GSP an edge-AI architecture as ideal as Blaize claims?
Once again, frustration and skepticism among industry observers lingers, because the startup hasn’t fully disclosed the technical details behind its GSP.
In the big picture, Blaize maintains that its GSP architecture is built on a graphstreaming model. In contrast, legacy processors such as GPUs/CPUs and FPGAs are based on a batch processing model. The inherent advantage of graph streaming processing is its ability to offer task-level parallelism, while processing data in real time.
However, graph streaming, not exactly a ground-breaking idea, has been around for many years. It was deployed by now-defunct Wave Computing. Now the U.K.-based AI unicorn company, Graphcore, is also using it.
So, how to compare Blaize GSP with Graphcore’s graph streaming, for example?
Omdia’s Kaul said, “Yes, graph streaming itself is not a new concept. We have yet to have a briefing on the technical details of graph streaming and so I cannot [comment] further on their differentiation.” Nonetheless, the startup overall, “seems to be one of the few showing some commercial traction with graph flow processing at the edge,” said Kaul.
Moor Insights & Strategy’s Freund noted, Blaize “hasn’t revealed a lot but the GSP is probably similar to the Graphcore IPU in that cores access local SRAM memory for weights and activations.” He added, “The Graphcore solution is primarily targeting training (Big chip, floating point, etc) while Blaize is targeting low-power and low-cost edge apps.”
Rajesh Anantharaman, Blaize’s director of products, told EE Times that he’s skeptical that Graphcore’s processor can represent graphs natively throughout its entire process. In contrast, he noted, when Blaize’s hardware, using OpenVX to accelerate computer vision applications, for example, presents graphs completely.
Bottom line
Lack of detail about Blaize’s GSP architecture is keeping some analysts on the sideline.
While Blaize hasn’t named its customers (other than its partner Denso), Omdia’s Kaul, for example, believes Blaize is starting to get traction with customers who pose large volume potential.
Similarly, given Blaize’s one-year market lead with test chips, Freund said, “I would not be surprised to see at least one of these trials go into production by early next year.”
Will Blaize become a real contender against Nvidia and Intel (Movidius and Mobileye)? Neither analyst will commit.
Asked what he would recommend if a car OEM asked for his advice, Freund said, “If you want a mobile Datacenter approach that can do it all, use Xavier. If you want a low-cost solution, say for driver monitoring, use Blaize.”
In the end, “Most OEMs will opt for a one-stop-shop and use Xavier,” Freund conceded. Running against incumbents is always tough. Blaize’ Munagala, nonetheless, believes that he’s done enough homework to meet “the unmet needs” of the edge AI market.
The article originally published at EE Times.
Advertisement