Remaining competitive in the Industry 4.0 era means manufacturers must be nimble enough to engineer for choice and be able to alter production processes to meet varied customer preferences or unique requirements. At the same time, maintaining this level of factory control is a challenge because even seemingly minor reconfigurations in factory equipment—something as seemingly innocuous as swapping out one sensor for something nominally equivalent—can have major consequences. The answer to both problems is greater flexibility with software control, enabling software-defined factories.
Our current issue is that manufacturing equipment has yet to be designed to deal with the high levels of product variance that we see today. Product quality is another important element to consider, especially in the Industry 4.0 era of predictive maintenance to optimize processes and maximize machine uptime. To achieve higher flexibility within the future smart factory, this article explores the potential of the software-defined factory that combines reconfigurability with enhanced accuracy and precision, as well as advanced diagnostics.
The benefits of measurement at the edge
The prevailing trend in modern factories is digitalization, but we still live in an analog world with all of its physical properties, such as temperature and pressure, and this information must first be acquired before being processed further. As a general rule, the measurement will be more accurate the closer the analog signal is converted to a digital signal. That is because it eliminates the possible parasitic resistances, capacitances and inductances that long cable runs would introduce, impacting the accuracy of the measurement.
The most dependable method to satisfy the needs of measurement and processing is an analog front end (AFE) for the precise capture of analog data right at the edge. The basic building blocks of a generic AFE are shown in Figure 1.
The AFE sits in between the analog sensor that measures a particular form of energy and the microcontroller, which processes the digital equivalent of the sensor’s data. It performs multiple functions, such as amplifying weak signals, filtering out unwanted noise, converting from one signal type to another and monitoring the signal for performance drift.
Even though factories have become smarter over time, a simple sensor failure can cause havoc. Figure 2 illustrates a factory floor with various conveyor belts, robots, control stations and so forth operating under normal working conditions.
If we consider a scenario in which one of the sensors fails, it stops sending a signal to the programmable logic controller (PLC).
The obvious course of action is to stop the machine and replace the sensor, but we all know that lately, we’ve had a lot of supply shortages. And what if this particular sensor is back-ordered? Production could be halted for several weeks—and costs associated with downtime are high. According to Forbes, unplanned downtime costs industrial manufacturers as much as $50 billion a year.
A possible solution to avoiding this prolonged downtime would be if the sensor manufacturer offers the factory a similar sensor, but there is a possibility that it comes with a different output. If that were the case, the maintenance team would have to replace the PLC and/or the remote analog input module.
Looking across the entire factory floor, for every sensor type in use, there could be several AFE versions. Most AFEs on the market accept a certain type of analog input, such as voltage, current or resistance, and transfer it into the digital world.
For example, an AFE could be set up to receive input from a temperature sensor, which in most cases is a very small voltage, to convert the analog signal into a digital signal. Another AFE could be configured to receive a voltage input, which could span 20 V, a scenario quite different from the AFE measuring the small voltages of a temperature sensor.
Benefits of universal AFEs
To solve this issue of having to change the AFE each time, the industry has developed universal AFEs that can accept analog inputs from a variety of different sensor types. So if the universal AFE measures voltage one day and the sensor fails but the replacement sensor has a current output, the AFE can be programmed to measure current instead.
The next logical step is to create a software-configurable universal AFE. An example is NXP’s N-AFE. In comparison with other universal AFEs currently available, it also integrates all of the discrete components that go into the system, such as protection and signal conditioning, and a slew of diagnostics features. Because it is software-configurable, all the maintenance engineer has to do is replace the sensor and reconfigure the analog input module remotely via the user interface, eliminating the time required to replace the entire measurement unit.
In a system that integrates universal AFEs (see Figure 3), the role of the PLC is expanded to include performing safety checks before, during and after these actions by communicating with internal diagnostics and programming terminals to ensure that everything is running smoothly.
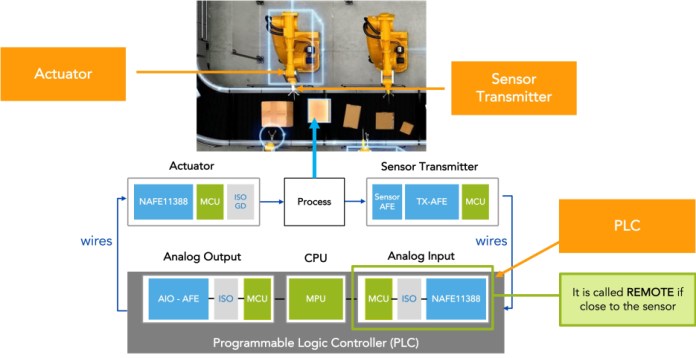
Figure 3: Software-configurable universal AFEs enable swift and easy changes to equipment on the factory floor. (Source: NXP Semiconductors)
Maintaining quality
Product quality is another important element in the industrial world, especially in the Industry 4.0 era. In addition to reducing the complexity of the system solution and bill of materials cost, NXP’s N-AFE is more precise and accurate.
All measurements tend to be made with respect to an internal reference, which is integrated within the AFE. For most devices on the market, the quoted specifications are with respect to an “ideal” reference and do not necessarily consider drift and hysteresis over time. However, NXP’s universal AFE will store the internal reference in non-volatile memory. If the internal reference does not correspond to the stored value, compensations can be made, maintaining the AFE’s precision and accuracy over time.
Manufacturers are turning toward predictive maintenance to optimize processes and maximize machine uptime. This aims to boost production efficiency while streamlining manufacturing infrastructure management. For example, when bearings start to fail in a motor, the signature of the current changes, albeit very subtly.
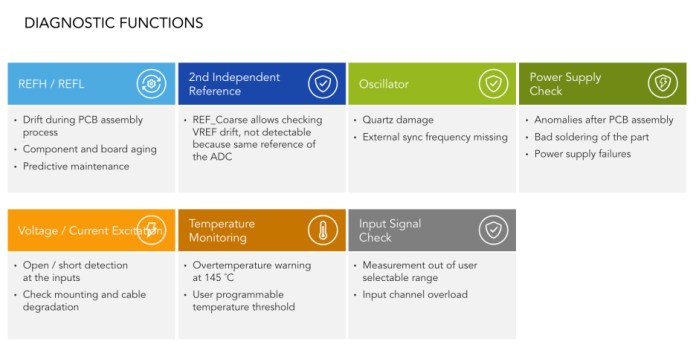
Table 1: Software-configurable universal AFEs can cover a range of advanced diagnostic functions, enabling swift and easy changes to equipment on the factory floor. (Source: NXP Semiconductors)
The increased accuracy of the sensor data and slew of diagnostic and anomaly-detection features unlock new levels of visibility and insight, improving preventive maintenance regimes. In addition, identifying issues before they occur increases factory uptime and efficiency and improves safety.
With the appropriate processing power and artificial-intelligence algorithms and models, machine operators can better judge whether the bearings are deteriorating to the extent that it will affect product quality. For example, in the pharmaceutical industry, very small variations in a measurement could compromise the proper dosage of a pill.
About the author
Alec Makdessian is marketing and business development director at NXP Semiconductors, managing analog ASSPs for industrial applications. He has 20 years of experience in the semiconductor industry as an applications engineer, as well as various marketing and management roles. Alec holds a B.S.E.E. and an M.S.E.E. from the University of Texas at Austin.
Advertisement
Learn more about NXP Semiconductors