Artificial intelligence and medical imaging have combined to create a revolutionary shift in the field of medical diagnosis. Medical imaging techniques, such as X-rays, magnetic resonance imaging (MRI) and computed tomography (CT) scans, are complemented and even superseded by AI.
In addition to improving diagnostic turnaround time, this integration opens previously inaccessible doors for tailored medication. One major development is the increased use of deep-learning algorithms, which can sift through massive datasets in search of abnormalities and patterns, providing a higher degree of previously unavailable accuracy.
Improving the precision and efficiency of image analysis is a major focus of current research in both AI and medical imaging. Radiomics is a new subfield of medical imaging that promises to revolutionize the way data is extracted from medical images.
In the past, doctors relied on skilled radiologists to spot abnormalities in imaging tests like X-rays, MRIs and CT scans. Diagnostic results are vulnerable to human error and weariness. AI with deep-learning capabilities can tirelessly study massive datasets and detect minute changes and irregularities that humans might miss.
With radiomics, not only is the diagnosis process sped up, but the potential for error is also reduced, leading to better patient outcomes. It also aids in gaining a more complete picture of a person’s health status. More precise and individualized treatment plans will be impossible to achieve without the capacity to extract latent information from medical images.
The algorithms that interpret intricate visual data are fundamental to AI in medical imaging. Continuous efforts to improve algorithmic precision, efficiency and interpretability constitute a key trend in this field. Convolutional neural networks (CNNs) have been instrumental in image-recognition tasks, and their application in medical imaging is becoming more complex.
Transfer learning, a technique in which pre-trained models are adapted for specific tasks, is gaining popularity, enabling the development of AI systems using relatively small quantities of medical imaging data. As algorithms become more refined, they improve diagnostic accuracy and help identify subtle patterns and anomalies that may elude human observation.
AI is not only being used to analyze and interpret images but also to streamline medical imaging procedures. Automating the scheduling of imaging analyses and maximizing the efficiency of imaging equipment are two key use cases for AI algorithms.
GE HealthCare, a leading innovative company in the field of medical technology and diagnostics, has developed the Definium 656 HD, a fixed X-ray system (Figure 1) that provides several AI-based capabilities. Using 3D camera technology, GE’s Intelligent Workflow Suite is designed to produce more consistent images and eliminate the need for retakes.
The system incorporates Version 2.2 of GE’s Helix AI-based advanced image-processing software, which provides enhanced anatomic detail and clarity regardless of dose, patient positioning, field of view or metal implants.
Siemens Healthineers AG, a pioneer in healthcare innovation, has developed several digital applications based on AI. One technology is Deep Resolve, which uses CNNs to speed up MRI scans (by as much as 70% in the case of brain imaging) thanks to AI.
The compromise between scan time, resolution and picture noise defines MRI quality. To improve one of these factors, there likely will be a tradeoff. Deep Resolve solves this challenge by enabling doctors to select a much shorter scan time without sacrificing picture quality by decreasing noise and maintaining or improving resolution. Deep Resolve utilizes the raw data from the scanner to enhance the image using AI algorithms during the early stages of image reconstruction.
Patients who are anxious in MRI scanners, such as young children, benefit from shorter scan times. The example in Figure 2 shows how Deep Resolve can reduce an MRI knee scan to less than two minutes, compared with about 10 minutes with conventional equipment.
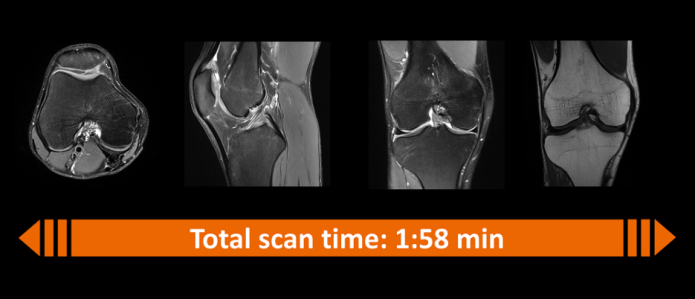
Figure 2: Deep Resolve reduces an MRI scan of a knee from about 10 minutes to less than two minutes. (Source: Siemens Healthineers)
AI-based medical project
Having the right medical imaging datasets is essential to ensure that AI and data science algorithms remain objective, even with the best hardware and software. Experts in the field of AI at Harvard Medical School have launched the Medical AI Data for All (MAIDA) project to collect and disseminate medical imaging datasets from all around the world.
This exhaustive dataset of patient radiology images will soon be available to the research community thanks to an international partnership initiative. Addressing the limitations of existing data resources, MAIDA will be a carefully curated, de-identified, diverse library of datasets containing pertinent clinical information and granular, high-quality annotations. Relevant image sets from 41 institutions in 17 countries have already been contributed to this collaborative effort.
The MAIDA project will initially focus on chest X-rays, which are frequently used in emergency rooms to rule out pneumonia and other diseases. However, other clinical use cases included in this program are endotracheal tube (ET) assessments in neonatal and adult intensive care units (ICUs). Incorrect placement of the ET tube, relatively frequent, can cause serious problems like low blood oxygen levels, high CO2 levels and a collapsed lung.
During the Covid-19 pandemic, the Terasaki Institute for Biomedical Innovation launched an AI-based project to develop testing for fast and accurate detection of Covid-19 infection. Traditionally, swab samples taken from the nose or throat are tested using a process called reverse-transcription polymerase chain reaction (RT-PCR). Sampling mistakes, a low viral load and the method’s low sensitivity all contribute to the potential for inaccurate results.
Lung images provide a complementary diagnostic tool for Covid-19. Chest radiographs and CT scans can be used to differentiate Covid-19 from other lung injuries and to evaluate the extent to which the lungs are affected by the virus.
Diagnostic capacities can be improved when the images are combined with AI models (Figure 3). Created using data from 704 chest X-rays, the model was confirmed with data from 1,597 additional cases, including healthy individuals and patients with pneumonia and Covid-19 obtained from other sources. The model delivered excellent results in classifying the diagnoses, according to the Terasaki Institute.
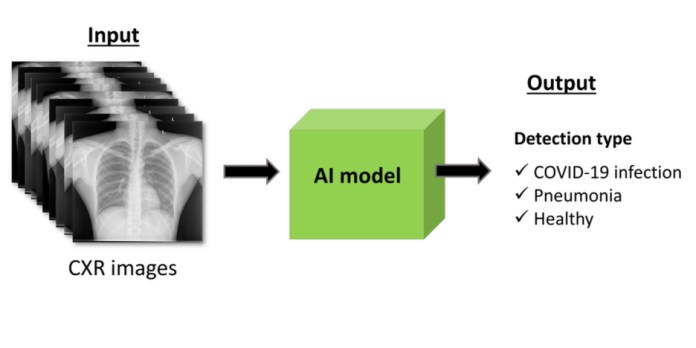
Figure 3: AI models help precisely detect Covid-19 and other lung diseases. (Source: Terasaki Institute)
Edge computing
Improvements in hardware have been one of the driving forces for the convergence of AI and medical imaging. AI algorithms for image analysis demand enormous amounts of computing, requiring dedicated hardware, such as FPGAs and microcontrollers, to effectively manage the complexity of deep-learning models. Constant improvements to these hardware components are increasing the efficiency of image analysis and making it possible to seamlessly incorporate AI solutions into the medical imaging infrastructure.
The implementation of edge computing is a significant change for AI in medical imaging. Traditional methods of analyzing medical imaging data involved sending massive datasets to a central server, which presented problems with latency and confidentiality.
To reduce latency and enable real-time analysis, edge computing processes data near its origin. In the field of medical imaging, this entails embedding AI algorithms within individual imaging machines. This not only improves the efficiency of healthcare delivery by speeding up the diagnosis process, but it also paves the way for point-of-care AI applications whereby choices can be made immediately.
Advertisement