The market for artificial intelligence in medical diagnostics will exceed $3 billion by 2030. By this time, the use of medical-image–recognition AI will have risen by nearly 3,000% due to its ability to analyze data much more efficiently than humans, according to IDTechEx’s report “AI in Medical Diagnostics 2020-2030: Image Recognition, Players, Clinical Applications, Forecasts.” This technology has the potential to refine diagnosis methods and minimize time to treatment by streamlining the image analysis process.

Image-recognition AI is a decision support tool that generates rapid and informative insights into a patient’s condition. (Source: IDTechEx) Click for larger image.
Since the introduction of deep learning in image-recognition software in 2010–2014, the market for AI-enabled image-based medical diagnostics has entered a state of rapid technological expansion. AI companies are continuously seeking to widen the range of capabilities and applicability of their product in order to strengthen their presence in this competitive market.
Major innovations in this industry revolve around more efficient use of data, increasing the accessibility of this technology, and enhancing its value proposition to radiologists. This article examines five key trends in image-recognition AI technology for medical imaging.
Moving toward superhuman disease detection
Accuracy is a primary concern when evaluating the value of image-recognition AI in medical diagnostics, and AI companies are striving to achieve superhuman levels of disease detection. Currently, despite AI’s potential to revolutionize the disease-diagnosis process, its current value proposition remains below the expectations of most radiologists.
Many AI companies are making the refinement of their algorithms a priority. For instance, Dutch startup SkinVision successfully improved the disease-detection performance of its software to bolster its credibility as a decision support tool. In 2014, the SkinVision app detected 81% of skin cancer cases, which was considered insufficient to detect melanoma accurately. By 2019, this figure had climbed to 95% to become the highest on the market — far above the 70% to 80% accuracy of human dermatologists.
Today, the level of accuracy of an algorithm is its No. 1 selling point, and companies that reach superhuman accuracy possess a crucial competitive advantage. Achieving this will facilitate the uptake of their technology in medical settings, as the benefit of automated actionable quantitative insights will outweigh the short-term inconvenience of changing the workflow.
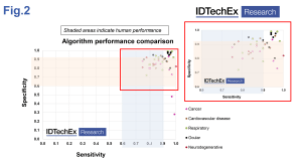
Image-recognition AI in medical diagnostics: performance comparison by application versus human performance (Source: IDTechEx) Click for larger image.
Widening the software’s applicability by increasing diversity in training datasets
AI companies are increasingly focused on expanding the applicability of their software. Currently, a common limitation of image-recognition AI algorithms is their restriction to a certain population type. As a result, the software’s ability to detect disease can be reduced if the patient profile does not match the data type that it was previously exposed to.
To address this issue, AI companies are including more diverse datasets during algorithm training. There is a growing consensus that training data should encompass numerous types of patients so that the algorithms can recognize abnormalities regardless of patient ethnicity, genetic background, or physiology. For example, Lunit Inc.’s INSIGHT MMG software can detect breast cancer with 97% accuracy because the company trained its algorithms to recognize lesions in breasts of different densities and fatty tissue composition.
Demonstrating success in dealing with a wide range of patient demographics represents a key technical and business advantage. It expands the applicability of the software, thereby helping to boost its usage across a wider patient demographic.
Ensuring high image resolution to maximize algorithm performance
Obtaining high-resolution images is key to maximizing the disease-detection performance of AI and increasing the reliability of AI-generated insights. The use of poor-quality data during training negatively impacts the development process and performance levels of deep-learning algorithms. Unclear images reduce the accuracy of insights generated by AI, which can damage its chances for widespread implementation.
In response to this, AI companies are developing methods to enhance the value of image-recognition AI in medical settings by capturing better images. AI-driven tools for assessing or improving image quality are already commercialized. For instance, U.S.-based Subtle Medical Inc. uses image-recognition AI to transform blurry images unsuitable for analysis into high-resolution scans.
Another approach is used by India-based Artelus, which has developed a system that assesses image quality immediately after acquisition. This system determines whether the image is sufficient for reliable diagnosis or if the image should be retaken.
Detecting multiple diseases from a single image
The detection of multiple diseases is another important trend in the image-recognition AI space. In the past, companies preferred to focus on the detection of a single disease, as it is much less costly and time-consuming. As a result, many AI-driven analysis tools today can identify only a restricted range of pathologies. Their value in radiology practices is hence limited, as the algorithms may overlook or misconstrue signs of disease that they are not trained on, which could lead to misdiagnosis.
To increase the appeal of their product to doctors seeking versatile decision support tools, AI companies are allocating more resources to recognizing not just one but various conditions from a single image or dataset. For example, solutions from DeepMind Technologies and Pr3vent Inc. are designed to detect over 50 ocular diseases from a single retinal image, while VUNO Inc.’s algorithms can detect a total of 12.
For both hospitals and AI companies, software capable of detecting multiple pathologies offers much greater value than software detecting a specific pathology. Because one scan is sufficient to detect a myriad of diseases, health-care costs can be kept to a minimum by reducing the number of patient examinations required to reach a conclusive decision. The wider applicability of multi-disease–detection software also enables it to be used more routinely within hospitals as a go-to diagnosis tool.
Integrating AI software into imaging equipment
Integrating image-recognition AI software directly into medical scanners is becoming more common. Currently, radiology AI software is generally deployed via cloud-based platforms or installed directly into the hospital’s internal servers. This reduces productivity as radiology practices adjust to a new workflow and has been known to discourage hospitals from adopting image-recognition AI.
AI companies are increasingly open to integrating their software directly into scanners in order to facilitate the automation of medical image analysis. The enhanced analytical capabilities provided by the AI software enable hospitals to maximize the number of patients seen every day and improve patient outcomes. This is being done more and more. Recent examples include Lunit Inc.’s INSIGHT CXR integration into GE Healthcare’s Thoracic Care Suite and MaxQ AI’s Intracranial Hemorrhage (ICH) technology being embedded into Philips’s computed tomography systems.
Advertisement
Learn more about IDTechEx