Maxim Integrated has released its first AI chip, the MAX78000 low-power neural network accelerated microcontroller (MCU) that enables artificial intelligence in battery-powered IoT devices. The MAX78000 SoC integrates a dedicated neural network accelerator with two microcontroller cores – the ultra-low-power Arm Cortex-M4 and lower power RISC-V.
Thanks to a novel architecture, this new breed of AI MCU enables neural networks to execute at ultra-low power.
“This is the first major implementation that makes use of the ultra-low-power processing capabilities of the Arm M4 coupled with a neutral network accelerator to best address the needs of AI in IoT while maintaining the low-power consumption envelope,” Kelson Astley, research analyst at Omdia told Electronic Products.
Although Astley couldn’t comment on the overall cost of the device, he said “as far as the ability to push this level of inferencing, at such a low power consumption cost, further out to the edge is the proof of the AI edge dream.”
Maxim focused on four key challenges – energy, latency, size, and cost – in the development of this architecture for AI in battery-powered IoT devices. The result is a device that can execute AI inferences at less than 1/100th the energy of software solutions, which significantly improves run-time for battery-powered AI applications, while enabling complex new AI use cases previously considered impossible.
Maxim said these power improvements don’t compromise in latency or cost. The MAX78000 executes inferences 100x faster than software solutions running on low power microcontrollers, at a fraction of the cost of FPGA or GPU solutions, said Kris Ardis, executive director for the Micros, Security and Software Business Unit at Maxim Integrated.
This is a game changer in the typical power, latency, and cost tradeoff, he added. He calls it “cutting the power cord for AI at the edge,” enabling battery-powered IoT devices to do much more than just keyword spotting.
“I think it will drive an expansion of more low-cost, low-power implementations that make use of this more general-purpose MCU instead of being relegated to high-cost ASIC SoC solutions. I see this being expanded out to remote industrial monitoring & tuning (oil & gas) as well as urban infrastructure monitoring IoT,” said Astley.
Addressing the AI ‘gap’
Bringing AI inferences to the edge meant gathering data from sensors, cameras and microphones, sending that data to the cloud to execute an inference, then sending an answer back to the edge. This architecture works but is very challenging for edge applications due to poor latency and energy performance, said Maxim.
An alternative is low-power microcontrollers that can be used to implement simple neural networks; however, the challenge is latency and only simple tasks can be run at the edge.
The MAX78000 is designed to fill this gap. “When we think about IoT and we think about all the battery-powered things around us, those [big FPGA or GPU AI] processors can’t run on the battery, they’re too big for a lot of the IoT devices, and they are not at a cost point where it makes sense to deploy,” said Ardis.
“What could we do if we could close that gap? You can think about some of the things that might affect you or me, such as cameras that could have smarter warnings by doing a better job of analyzing what they see, such as in battery-powered cameras,” he said.
Artificial intelligence could help with smarter analysis of these images such as identifying if there really is a person walking or if it’s a squirrel running outside. Other possible uses include better spatial awareness, or better vision, and deeper vocabulary spoken commands for small devices such as hearing aids or headsets.
“The exciting thing about AI technology is there are so many things that we can’t even imagine yet,” said Ardis. “But with this AI revolution there is a big gap,” he explained.
Ardis calls it a gap between big machines and little machines. The big machines are what we’re used to talking about, such as self-driving cars. “That’s an unconstrained universe where they have infinite power supplies and infinite cost budgets, and no size constraints.”
But the embedded devices that are sitting on your desk right now or in your house, AI hasn’t reached that so much, he said. “We see simple things like wake words, but we don’t really see a whole lot of AI, so there is this gap between these two universes.
“Our goal is to let those type of devices start to hear and see more of the world around them, like those big machines are doing today. But there’s a problem with trying to do that. The reason why the big equipment with infinite power supplies can do it today is because the workhorse of artificial intelligence, especially deep learning, is the convolutional neural network. These networks are basically a mathematical structure for deciding whether that picture is a car or a cat or whether you heard a certain word,” explained Ardis.
He said these neural networks do a great job of identification but they are very computationally expensive. “So you have very powerful and expensive power-hungry processors that that can make quick work of this stuff.”
For IoT devices it’s going to need to add that intelligence without adding any bulk and then there is the cost, said Ardis. “The cost points of current AI solutions are okay for expensive devices, but not for widescale IoT types of deployments.”
The solution
Leveraging Maxim’s expertise in wearables and low-power IoT applications, and “maniacal focus on energy and in particular reducing energy consumption for edge devices,” the company has a lot of experience making complex hardware to solve tricky problems, especially tricky problems that require a lot of computation, said Ardis. “We have a very long history of making smart and complex crypto engines to reduce latency and energy for those types of computations.”
In addition, Maxim understands how to build and integrate the right functions for the smallest possible end products. “That integration approach is letting us get to cost points that will enable more of that mass deployment of embedded AI because we’re coming from a lower end embedded AI solution compared to the kind of GPU-type devices that are available today.”
The MAX78000’s combination of dedicated neural network accelerator with two microcontroller cores enables machines to see and hear complex patterns with local, low-power AI processing that executes in real-time. This translates into much higher power efficiency for applications such as machine vision, audio, and facial recognition.
“The two micros aren’t actually involved in the neural network computations and the AI inference itself except to configure the accelerator and load the data,” said Ardis. “One of the reasons why we use the RISC-V is because it is a lower power core. It’s in the same power domain as that accelerator and when a customer is trying to squeeze the last amount of energy out of the system they would put that kind of lower level code on the RISC-V to move data from the outside world into the accelerator.”
The centerpiece of the MAX78000 is the neural network accelerator, which is designed to minimize the energy consumption and latency of convolutional neural networks (CNN). This hardware runs with minimal intervention from any microcontroller core, and power and time are only used for the mathematical operations that implement a CNN. Designers can use one of the two integrated MCU cores to move the data from the external world into the CNN engine.
“The accelerator is purely our design. It has the memories it needs to store the network configuration, a fair amount of data memory for whatever image or sound that will be stored on-chip, and it’s highly flexible so engineers can use conventional tools like TensorFlow and PyTorch, which is really what most of the machine learning universe uses today to train networks for this accelerator.”
“The data is where we get the biggest bang for the architectural buck to minimize the overall energy system spend,” said Ardis. “Another thing that helps is that no external memory is needed. There are limits to what we can do but the tradeoff is 100× or even 1000× lower energy.”
Running the same exact neural network versus one of our low-power MCUs, we’re over 1000× lower energy on MNIST, 600 times lower energy on keyword spotting, and that is without optimizing the network, he said. “You can either reduce your battery size by a significant amount if you’re already using this kind of machine learning technology today or you can enable new use cases that you couldn’t even approach before.”
The neural network accelerator also offers flexibility in power tradeoffs, so engineers can customize their energy spend. While the accelerator is optimized for energy, engineers have the flexibility to run faster on higher current and go to sleep faster or run slow at a lower current thanks to flexible clock control.
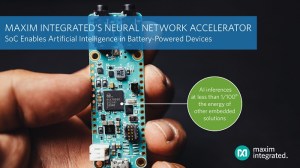
MAX78000 low-power neural network accelerated chip moves AI to the edge without performance compromises in battery-powered IoT devices.
In terms of size, the MAX78000 is housed in an 8 x 8-mm BGA package and is less than half the size of any GPU or big processor AI solution. Maxim has the capability to go to a wafer-level package, which will cut the size down by another factor of four, said Ardis.
By only requiring a few external components, it also makes placement and procurement easier for implementing vision on edge devices a much more cost-effective solution than what’s available today, he added.
The MAX7800 is available from Maxim’s authorized distributors. An evaluation kit, the MAX78000EVKIT#, is available for $168. The kit includes audio and camera inputs, and out-of-the-box running demos for large vocabulary keyword spotting and facial recognition. Documentation helps engineers train networks for the MAX78000 in the tools they are used to using: TensorFlow or PyTorch. Here’s a video to get you started with the MAX7800EVKIT# evaluation kit.
Advertisement
Learn more about Maxim IntegratedMaxim Integrated Products