When it comes to the dynamic world of technology, generative artificial intelligence is one of the most significant advancements. We are rethinking creativity, problem-solving and even interactions between humans and computers because of this disruptive technology.
Many sectors are experiencing profound changes because of generative AI, which is driven by state-of-the-art machine-learning (ML) models. These sectors range from semiconductor design and healthcare to content production and banking and beyond.
This article provides an overview of the current landscape of generative AI, examining its challenges, applications and future directions. The focus is on understanding the capabilities and limitations of generative AI, its impact on various industries and ongoing research trends.
Understanding generative AI
Generative AI is a subset of AI that aims to create original content from scratch instead of merely analyzing or recognizing specific patterns. Artistic expressions in any form, including text, images, sound, 3D models or even code, are fundamental to this technology. Generic AI uses neural networks trained on extremely large datasets to produce unique outputs, as opposed to conventional AI models that depend on rules or necessitate substantial programming.
The disruptive potential of generative models is what makes them significant in many different fields. Generative adversarial networks (GANs) are examples of generative models. GANs are a category of ML models specifically engineered to produce authentic and novel data instances that bear a resemblance to a provided dataset or training data.
GANs are based on the concept of two neural networks, a discriminator and a generator, participating in a game-like scenario. As input, the generator network acquires the ability to produce data that closely resembles authentic data through training on a given dataset. As it evaluates input data, the discriminator network attempts to differentiate between authentic data extracted from the dataset and generated synthetic data. Training is conducted to enhance the model’s capacity to distinguish between authentic and generated data.
While standard ML models aim to classify or predict data, generative models try to understand the dataset’s distributed features. They can imitate current facts and create new data or content thanks to this ability, which encourages innovation and creativity.
Multimodal generative AI
Directly related to generative AI is multimodality, which entails the capacity to process inputs from multiple senses to generate outputs in either identical or dissimilar data formats. Video, audio, speech, pictures, text and various conventional numerical datasets are all used and trained with multimodal AI systems. Most crucially, unlike prior AI, multimodal AI makes use of a wide variety of types of data simultaneously to aid with content development and context interpretation.
The algorithms that govern the learning, interpretation and response-building processes in artificial intelligence are known as AI models. After the model consumes data, it trains and constructs the neural network that underlies it, setting a standard for appropriate answers. The AI learns from the user’s input and uses that knowledge to make decisions and provide responses. That result, along with any other incentives the user may have, is sent back into the model for further improvement.
In conventional AI, datasets are the primary differentiator between multimodal and single-modal ML. The default setting for single-modal AI is just to support that one data type or source. Contrarily, multimodal AI draws on a wider variety of inputs, such as visuals, audio, speech and text, to provide richer and more complex views of a given setting. By doing so, multimodal AI brings its perception closer to that of humans.
The risk of hallucination
AI hallucination occurs when a large language model (LLM), such as a generative AI tool, sees patterns that do not exist or are not visible to humans, leading to illogical or incorrect outputs. This means that AI generative algorithms are not always going to reliably produce results that are based on training data, are accurately interpreted by the transformer or exhibit any discernible pattern. Put simply, the answer is “hallucinated” by it.
A transformer is a unique neural network setup that has shown impressive effectiveness when dealing with tasks involving sequential data, such as natural language processing (NLP). When it comes to generating text, generative AI models rely on the transformer architecture because of its ability to handle context, dependencies and relationships within a sequence of words. These models, which have been trained on extensive amounts of text data (such as GPT), can produce coherent and contextually relevant text based on the input they receive.
Three of the main causes of these misunderstandings in AI are the following:
- Overfitting: It is an undesirable characteristic of ML wherein the model produces accurate predictions exclusively for the training data and not for the new data.
- Biased or inaccurate training data: An AI model that has been trained using a dataset containing biased or unrepresentative data may experience hallucinations of patterns or features that are indicative of these biases.
- Excessive model complexity: The probability distribution of a model determines the likelihood it assigns to different outcomes. A wide probability distribution indicates that the model can assign reasonable probabilities to a wide range of possible outputs. On the other hand, if the model is overly complex and lacks sufficient training data, it may result in a narrow probability distribution. This means that the model becomes overly confident about certain patterns or generates outputs with high probability that do not accurately represent the true data distribution.
AI hallucinations may have severe repercussions for practical implementations. A healthcare AI model, for instance, may erroneously provide an incorrect diagnosis, resulting in unwarranted medical interventions. AI hallucinations may also aid in the dissemination of false information.
The best ways to mitigate hallucination risks are using only high-quality training data, introducing constraints that limit possible outcomes and ensuring, as a final step, that an individual is examining and validating AI outputs.
Use cases for generative AI
Natural language processing
NLP stands out as a prominent domain where generative AI has been implemented. LLMs like GPT-3 and GPT-4 have exhibited unparalleled capabilities in both language comprehension and generation. These systems can generate coherent and contextually pertinent text, respond to intricate inquiries and even participate in dialogues that closely mimic human interaction. The implications of this innovation for language translation services, automated customer support and content creation are substantial.
Semiconductor industry
Generative AI can be used to accelerate chip design. Synopsys Inc., a leading company in semiconductor electronic design automation (EDA), has recently introduced Synopsys.ai Copilot, a tool that integrates Microsoft Azure OpenAI Service and brings the power of generative AI into the design process for semiconductors.
Integrated into the complete Synopsys EDA infrastructure (Figure 1), Synopsys.ai Copilot is claimed as the first generative AI chip design capability. In its early stages, Synopsys.ai Copilot will function as a knowledge query system, extracting information from various Synopsys resources, including application notes, product user manuals, videos and documentation.
As it continues to learn and be enriched by your unique workflow and methodologies in a secure, closed-loop fashion, Synopsys.ai Copilot will develop the capability to generate workflow scripts and offer prescriptive guidance and recommendations.
Healthcare
The healthcare industry is also experiencing the effects of generative AI, specifically in the areas of medical imaging and drug discovery. AI models possess the capability to analyze medical images with an unprecedented degree of accuracy and velocity, thereby facilitating the early detection and diagnosis of diseases. Generative AI is assisting scientists in drug discovery to identify and predict the effects of potential compounds, thereby accelerating the development of novel treatments.
Recently, Genentech and Nvidia announced a partnership that seeks to revolutionize the process of discovering and developing new medications by collaborating on the optimization and acceleration of Genentech’s proprietary algorithms. Genentech, a subsidiary of the Roche Group, is an innovator in the use of generative AI to discover and develop novel therapeutics that more efficiently deliver treatments to patients.
Together with Genentech, Nvidia will accelerate these AI models on DGX Cloud, a platform optimized for the deployment of AI models suitable for industrial use. By integrating Nvidia’s BioNeMo (Figure 2) cloud application programming interfaces into computational drug discovery workflows, Genentech plans to use the generative AI platform for drug discovery that empowers biotech firms to scale model customization.
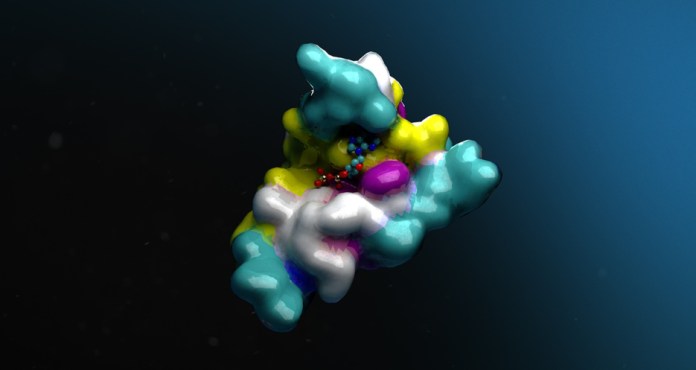
Figure 2: The BioNeMo cloud service helps to accelerate drug discovery and protein engineering. (Source: Nvidia Corp.)
Automotive
The automotive industry is poised to see an increase in the integration of AI, as generative AI progressively revolutionizes the cockpit environment. Already, conversational digital assistants are capitalizing on this emerging technology to establish more personalized connections between automakers and their customers and deliver in-cabin experiences that are unlike any other.
For example, SoundHound AI Inc. and Qualcomm Technologies are collaborating on the Snapdragon Digital Chassis concept vehicle to showcase the benefits of generative AI integration via the incorporation of SoundHound Chat AI for Automotive (Figure 3). By utilizing this platform, both passengers and drivers can pose virtually any inquiry and obtain a reply from a system that arbitrates intelligently to prioritize the most pertinent information from over 100 domains of knowledge.
This new demonstration, powered by the Snapdragon Digital Chassis and scalable AI-based Snapdragon Cockpit Platform, will enable free-flowing conversational queries by leveraging the Qualcomm AI Stack and industry-leading performance-per-watt hardware in conjunction with SoundHound’s edge and cloud products.
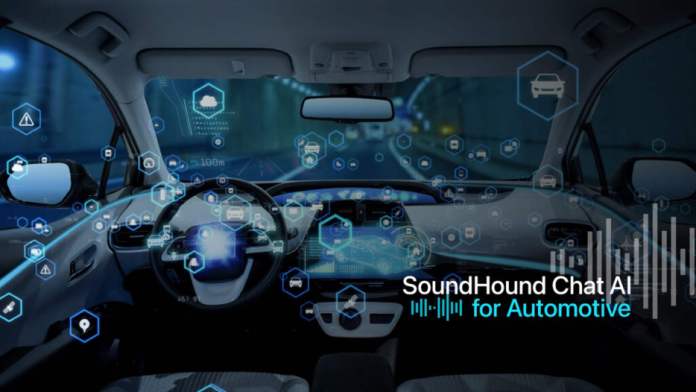
Figure 3: SoundHound Chat AI for Automotive will offer an in-vehicle voice assistant that combines generative AI capabilities with a best-in-class voice assistant. (Source: SoundHound AI Inc.)
Finance
Generative AI exhibits practical applications beyond the realms of science and creativity, extending into the sector of finance as well. Vast quantities of financial data can be analyzed by AI models to generate insights, forecast future events and optimize investment strategies. Rapid processing and interpretation of complex financial data provides a competitive advantage to financial institutions and facilitates more informed decisions.
Advertisement
Learn more about GenentechNVIDIAQualcommSoundHound Inc.Synopsys